INTRODUCTION
Cluster randomized trials are experiments in which unit of randomization are not individuals as in conventional randomized controlled trials, groups of subjects, social units or clusters rather than individuals are randomly allocated to treatment groups.1 Although, the standard approach is to randomize individuals to intervention and control groups yet certain health interventions, for example in nursing and public health are often implemented at the levels of health services, organizations and geographical area.2 Cluster randomized trials are increasingly being utilized in the evaluation of healthcare interventions as individual randomization is not always possible.3 There have been trial scenarios when the only possible approach for randomization is to randomize group of individuals. However, the associated complexities with the design and management of cluster randomized trials have been appreciated by different authors. Campbell et al, 20044 reported that, when compared with individually randomized trials, cluster randomized trials are more complex to design, require more participants to obtain equivalent statistical power, and require more complex analysis. This perhaps is responsible for the poor conduct and reporting of this design which have been noted by some authors.
MERITS, DEMERITS AND STATISTICAL ISSUES
One of the reasons of preference for cluster randomization in certain trial situations is to avoid the threat of contamination of some interventions which might result if individually randomized trials were to be used.4 At such times, groups of individuals, communities, families, schools, clinics, hospitals or medical practices are randomly assigned to treatment or intervention groups. For example, in a trial for the prevention of coronary heart diseases, factories were chosen as units of randomization to minimize the likelihood of subjects in different intervention groups sharing information concerning preventive advice on coronary risk factors. Even in cluster randomized controlled trials, researchers have taken measures to minimize the risk of having trials been contaminated. For example, in their study, Jafar et al5 ensured a distance of at least 10 km between selected clusters and if a selected cluster did not fulfil this criterion, it was dropped and a new one was selected.
Another reason for cluster or group randomized trial rather than an individually randomized trial is feasibility; it might be the only possible method of conducting the trial in some settings.4 For example, to evaluate a programme to enhance the effectiveness of hypertension screening and management in general practice. Such a program may not function well if there were some patients in the practice but not others were entered into it, in this case, the unit of randomization is the physician practice or the health facility. Interventions programmes that use mass education will usually make use of cluster randomized design as it might be difficult to educate a part of a community on certain health related issues, leaving the others. For example the effect of mass education on issues related to smoking cessation, dietary change or even exercise might be better captured by randomizing aggregates—communities, families, rather than individuals.
However, there are a number of drawbacks to this design method, which perhaps outweigh the merits. A foremost statistical problem is that, data analyses are not straight forward; cannot be done as if individuals had been randomized to treatment arms.6 There will usually be more to variability in individual responses both within and between the clusters; and hence, this must adequately be taken care of, to have a robust estimate of effect. For example, measurement error may vary in general practice, just as individual differences may also vary within and across clusters. The structure of variance both within and between clusters is perhaps responsible for the underline difficulty this design poses with respect to; sample size requirement, power, and test of significance of effect. In fact, Matthew6, further observes that, the effect of clustering actually increases the variance of the sample mean by a factor and if all the clusters have the same size, then,
clustering effect or design effect=1+ρ(m-1)
Thus, a modification in sample size (nc) to detect mean difference that takes clustering effect into account is given as:
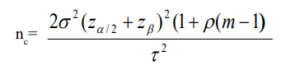
Here, σ represents the standard deviation, Zα/2 is the critical value of the normal distribution at α/2 – at 5% level of significance the critical value is 1.96, Zβ is the critical value of the normal distribution at β–for a power of 80%, β is 0.2 and the critical value is 0.84 and τ represents expected treatment effect. Depending on the cluster size (m) and the within-cluster correlation (ρ), clustering effect may have either increasing or decreasing effect on the standard sample size needed to detect a difference. The implication of this is that, there is a tendency to under-estimate the study especially at certain experimental conditions. This of course will affect both the precision and bias for estimating the effect. A more complex statistical approach is often necessary to adequately account for the clustering effect. In addition, the computation of the sample size here also places further responsibility on the investigators, as they need additional information than usual at the design stage. Information on both cluster size and the within-cluster correlation for the proposed study is needed. Such information may require extensive experience of the area of application for a successful trial design.
TYPES
Apart from the study objective, the structure of cluster on important prognostic factors also determines choice of appropriate cluster randomized controlled trial. For example, when there is substantial evidence that the cluster might differ significantly at baseline in prognostic factor, following randomization, then, similar clusters are paired and the two clusters in the same pair are then randomly allocated to interventions—matched pair. A classical example is the trial designed to assess the impact of improved treatment of sexually transmitted diseases on HIV infection in rural Tanzania. Here, the authors7, having identified twelve communities for the trial, noted that, communities in certain location exhibited higher incidence of HIV infection than others at the initial stage. The investigators, thereafter, formed six pairs from the communities, matched with respect to their location and a number of other factors. Each member of a pair was randomly allocated to the interventions following a baseline survey. It is worth noting that, intervention consisted of establishment of a sexually transmitted disease reference clinic, staff training, regular supply of drugs, regular supervisory visit to health facilities, and health education about sexually transmitted diseases.
Another design approach to a cluster randomized controlled trial and which perhaps could be more suitable when randomization into treatment arms involves large numbers of clusters is the completely randomized cluster design. This was the case in the study to evaluate the impact of vitamin A supplementation on morbidity: a randomized community-based intervention trial. Here, the authors8 randomized a sample of 450 villages into two treatments arms; the treated (vitamin A) group and the control group. During the course of the trial, each village was visited twice; for baseline data and a post-treatment assessment visit after a year. The third design approach is that in which similar or homogeneous strata are grouped together and are later randomized into treatment arms. Here, the allocation strategy resembles that which obtains for stratified randomization for individuals. The investigators stratify sample clusters for such factors that might have certain influence on the outcome. For example, the child and adolescent trial for cardiovascular health by Luepker et al9 , adopts a stratified cluster randomization to assess the outcomes of health behaviour interventions, focusing on elementary school environment, classroom curricula, and home programmes for the primary prevention of cardiovascular disease.
Statistical Analysis
Statistical analysis of cluster randomized controlled trials is much of a challenge as its design. The conventional regression analysis will be inadequate to evaluate the effect of the intervention as it is unable to capture the effect of other known sources of variation associated with the outcome measure, particularly the clustering effect. Statistical analysis that account for clustering effect is the most appropriate in this context. Statistical procedure options relevant to analyzing cluster randomized trials will be considered in subsequent notes. Meanwhile, interested readers may consult the following articles Campbell et al10, Murray et al11 as they provide useful information on such options available for statistical analysis of cluster randomized controlled trials.
CONCLUSION
Designing a cluster randomized controlled trial offers solutions in trial scenarios where it would be inappropriate to randomize individuals into treatment arms, its design is not without certain peculiar challenges. Part of the challenge is to be able to understand research environments when it becomes the only appropriate design option. The understanding of various types and the associated intricacies with each of them will in no doubt facilitates a correct design of the experiment.